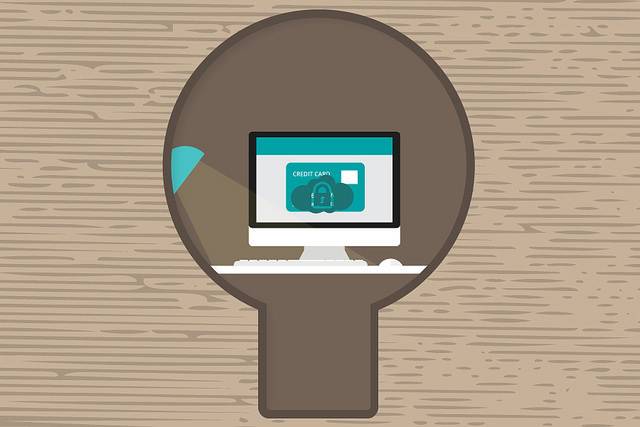
Machine learning is transforming computing on many levels, though much of the developments are hidden behind the scenes. E-commerce is seeing benefits from machine learning too, with enhanced fraud prevention one of the most promising developments. We’ll take a look at what machine learning contributes to fraud detection, and how accurate fraud detection boosts the profitability of e-commerce operators.
The way forward
Card not present(CNP) fraud is a problem for online merchants in particular, as customers are by definition physically not present at the point of sale. The problem is increasingly growing, with a huge rise in US CNP fraud: the US Payments Forum predicts a rise in CNP fraud to $6.4 billion in 2018.
Yet as much as it is key to put in place advanced fraud protection, ecommerce merchants need to be careful not to lose customers and revenue in the attempt to prevent fraud. Machine learning is a great way forward as the decisions derived by machine learning algorithms are far more refined compared to manually programmed parameters.
So, the detection is more accurate, and this matters for two reasons:
- Preventing chargebacks
Rules that accurately detect fraud can prevent fraudulent transactions from occurring in the first place. In contrast, if a merchant allows a fraudulent transaction through unintentionally they are liable to a chargeback plus fees and penalties on top. Not only does the merchant lose the goods shipped, they also pay a penalty fee. Even worse, frequent chargebacks can lead to the loss of a credit card merchant account. Thus, it is important to educate yourself about how different credit card networks handle disputes, as the way American Express deals with chargebacks is different then the Visa claims resolution process.
Claim up to $26,000 per W2 Employee
- Billions of dollars in funding available
- Funds are available to U.S. Businesses NOW
- This is not a loan. These tax credits do not need to be repaid
- Reducing false positives
The flipside of preventing CNP fraud is the possibility of overreacting: in other words, merchants can be at risk of declining bona fide transactions. Though this rarely happens ona very large scale unless a fraud system is truly malfunctioning, even a relatively small margin of error can cause loss of profitability. Also, if individual customers frequently find they cannot transact with a merchant, they may never come back. The more refined rules used by machine learning-based fraud detection systems are much less likely to trigger false declines.
How machine learning boosts fraud prevention
Fraud prevention is an area that is ripe for the application of machine learning. The detection of CNP fraud is a tricky process: exact rules programmed in painstaking detail simply cannot take account of every possible exception to the rule. The end result is that valid, bona fide transactions are declined, which causes a loss of revenue.
To build a model, a fraud detection system that is built on machine learning is fed two sets of data which are identical in structure, except for the fact that one set is legitimate transactions, while the second set is made up of fraudulent transactions, drawn from fraud reports. A machine learning algorithm then compares the two datasets and from there builds the knowledge to distinguish between a valid transaction,and an attempt at theft.
The system also applies the model to live transactions, analyzing the parameters according to statistical weightings to conclude whether a transaction is likely to be fraudulent. False positives can be reported back tothe algorithm and the process is repeated over time, enabling the system to learn from mistakes, reducing false positives.
Advantages of detection systems driven by machine learning
Though the manual review of transactions can incorporate some of the advantages of machine learning, as humans also learn through experience, there are many reasons why automated, auto-learning detection systems are advantageous.
Verification speed:Automated systems can check a transaction nearly instantaneously, whereas verification by humans delays the fulfillment of an order. That said, automated verification based on unrefined rules is not a good choice as the number of false positives can be large.
Learning and scale:Machine learning and automated systems drivenby it thrive on large datasets. By partnering with a fraud prevention provider that has access to large pools of data a business can enjoy a highly tuned fraud detection system. Such a system will far exceed the capabilities of even the largest human teams, as teams cannot pool knowledge equally.
Consistency and efficiency: One less discussed issue around human transaction verification is the fact that humans do not always work accurately. To make mistakes is human, and repetitive tasks can lead to cognitive failure over time. As fatigue sets in, efficiency also reduces. An automated system does not suffer from the same problems: it performs consistently and does not suffer a drop-off in efficiency.
Sophisticated fraud management is critical for e-commerce
Merchants have long been stuck with making a difficult choice between declining too many transactions, and eagerly accepting transactions – but enduring frequent chargebacks, and a hit to their reputation. Fraud detection systems based on machine learning algorithms help to bridge this gap, reducing the chance of refusing a legitimate customer while keeping fraudsters at bay.